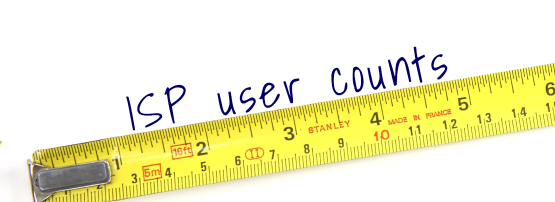
In our physical world census information is used to inform the planning processes behind the provision of infrastructure, such as schools, hospitals, housing, and similar. It can be used to assess the impact of natural disasters or to understand a society’s needs in terms of food and energy security. Demographic data is also used to inform investment and business decisions.
You’d think that the Internet itself would be awash with similar information. After all, much of the Internet’s economy is based on the aggregation of user profile data, repackaging this data, and selling it to advertisers in the form of advertisement (ad) placement capabilities. So, it’s likely to be the case that similar census-related data is being continually gathered in the Internet.
However, this data is a key commercial asset, owned by the corporate entities that gather the data. There is very little public data of a similar nature that relates to the market positioning of ISPs in terms of the number of users of their services.
In our measurement work at APNIC Labs, we’re trying to relate our measurement data, based on a sampled subset of users, to the larger picture of user populations. If you had information on the number of users for each ISP, it would be possible to derive data that could indicate the adoption levels of specific technologies, such as IPv6 or DNS security mechanisms.
This data would also be extremely useful in several areas. When a major ISP experiences a service failure what is the impact of service disruption where the ISP service has failed?
There was an eight-hour service outage experienced by a major ISP in Australia, Optus, on 8 November 2023. This provider is the second largest provider in the Australian ISP market, with an estimated 4M users, so the outage was a major incident.
The data would also be extremely useful in the area of public policy. How open is the market for the provision of Internet services within each economy? How many users are served by each ISP? What’s their respective market share?
Such information can also inform policy issues related to national security and resilience: How many local users are reliant on the services provided via a foreign platform?
Our response to this missing data set was to generate, on a daily ongoing basis, an estimate of the number of users per ISP for every ISP that we see on the Internet through the ad-based measurement platform. This report is published at APNIC Labs. As far as we are aware this is the only such public data set that encompasses the entirety of the public Internet.
In this post, I’ll explain how we calculate this data, and provide some responses to a recent presentation at the RIPE 89 meeting on this data set.
Data generation
The process starts with the estimated current population in each economy. The data we use is sourced from the United Nations Population Division. We use the mid-year population estimate from 2023 and apply the 2022-2023 growth rate to the period from mid-2023 to the present day to get an estimate of the current population of each economy for this day.
The second data set we use is the proportion of the population of each economy that is classed as Internet users. There are three possible sources for this data — the World Bank, the International Telecommunications Union (ITU) and the CIA World Factbook. We use the ITU data by preference, but the three data sets are well correlated in any case.
The combination of this data gives us an estimate of the current Internet user population per economy. It should be noted that this is not the number of ‘subscriptions’ to a service, as it attempts to include the number of users behind each subscription. It also is supposed to avoid ‘double counting’, so where a user is part of a broadband service and also has a mobile service, then the user is still only counted once as an ‘Internet user’.
The third component of the data is the ad presentation data of the APNIC measurement program. We use Google Ads to deliver some 25M individual ad impressions per day. We use the Maxmind geolocation database to map each user who received an ad impression to an economy and use a local default-free BGP routing table to also map each user to their ‘home’ network. At this point, we have now assembled a set of ‘home’ networks (origin Autonomous System Numbers (ASNs)) and the geo-located economy for each presented ad.
Assumptions
Here we make two major assumptions. Both assumptions are somewhat questionable, but we’ve been forced to make them in the absence of generally available data.
The first assumption is that Google’s ad placement algorithms apply to all users within a given economy uniformly. In defining the ad campaigns, we attempt to make the placement definitions as generic as possible, so that within each economy the ad placements are roughly equivalent to a random sampling drawn from all users in that economy. The implication of this assumption is that if an ISP has twice the number of users as another ISP in the same economy, then its users will receive twice the number of ad impressions. This could be stated as the distribution of ad placement and the distribution of users across ISPs are assumed to correlate.
The second assumption is that each user uses a single ISP for Internet access. This is not necessarily the case. For example, a user may use a local mobile service provider for their mobile Internet access and Starlink for their broadband access. We also have a user in their workplace using their workplace’s ISP and using a consumer ISP when they are at home. We are not able to account for such situations and in uniquely assigning each user to a single ISP in an economy we tend to underestimate the user count for each ISP in consequence.
The results we generate carry an inherent level of uncertainty due to the assumptions involved. Comparisons of this data with other sources, where we have access to ISP market share data for specific economies, suggest an overall uncertainty of about 20% in our estimates of users per ISP. Large consumer ISPs still show a high user population in the generated data, but the estimates for smaller networks are much less reliable.
The assumption of uniform distribution of ad placements across all ISPs within each economy tends to fail where the number of placed ads in relation to the per-economy user population is low. The best current example of this can be seen with the Russian Federation, where ad placement in this economy has plummeted since February 2023 (a consequence of the situation in Ukraine and associated Western sanctions).
The data for Norway reveals another assumption — that browsers do not use proxies. However, this assumption does not hold for Opera, which performs many data fetches on behalf of its users from its own servers. As a result, the system incorrectly assumes that AS39832, Opera’s ASN, is the largest ISP in Norway — about four times the size of the next largest ISP, Telenor. This Opera result is clearly inaccurate, and I should exclude Opera’s ASN from this dataset!
There is another assumption around the day of the week, and for holidays, where the analysis assumes that every day is much the same, whereas on business days the ad presentation into work-related ISPs is far higher than the presentation rate for the same ISPs in weekends and holidays.
As this is a measurement based on the placement of ads, the use of so-called ‘ad-blockers’ can disrupt this measurement. Our assumption here is that like the ads themselves, the use of ad-blockers is also relatively uniformly distributed across all users in the economy.
Conclusions
It’s frustrating that this information isn’t typically collected in annual filings for national regulatory agencies or compiled internationally by the ITU-T. This gap has motivated APNIC Labs to use our measurement data to publish our estimates as a public dataset. The conclusion from the recent RIPE presentation is that this method of estimation of the number of users for each ISP works well in economies with sufficient Google ad presentations, a conclusion that correlates with our own experience in running this measurement for many years.
On the other hand, the generation of this data is based on several sweeping assumptions, which I’ve noted here, and numbers should be treated with some level of caution.
The views expressed by the authors of this blog are their own and do not necessarily reflect the views of APNIC. Please note a Code of Conduct applies to this blog.