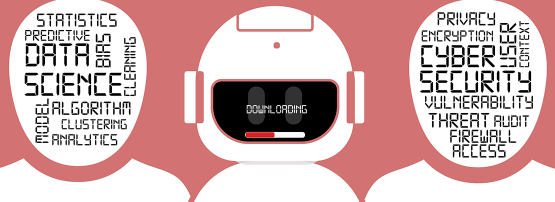
“Data is the new oil” has been a common phrase in business since it was first coined by mathematician Clive Humby in 2006.
Like oil, data, in its crude form, needs to be refined to be valuable; a process that can be painstaking and costly for even skilled statisticians. Data scientists have become increasingly sought after by various industries that are seeking to capitalize on this value.
This has certainly been the case in the cybersecurity sector, where Cybersecurity Data Science has emerged as an important and highly regarded profession to help prevent, detect, and remediate cybersecurity threats. It’s the evolving and dynamic nature of these threats that cybersecurity professionals are ‘hoping’ data science, combined with automation techniques (including machine learning), will allow them to react to and/or mitigate faster than they do today.
Although there is no definitive automated cybersecurity solution as yet, many businesses have published and presented case studies spruiking the potential of their techniques. However, most of this commentary is from cybersecurity professionals and vendors, with few observations (yet) from data scientists.
What brief commentary there is I’ve summarized below with comments from data scientist Dr Jeffrey Chan, Senior Lecturer at RMIT University, for the purpose of understanding the technical challenges of data science when it comes to automation, as well as the specific challenges of applying automation and data science to cybersecurity.
It quickly becomes clear that automation is not, nor will be, an out-of-the-box solution but requires a great deal of human expertise and resources both from the data science and cybersecurity fields.
Challenges of data science stem from its root
Two of the major challenges in applying data science in cybersecurity, or any industry for that matter are its multidisciplinary nature and the availability and interpretation of data.
Multiple disciplines and expertise help and hinder cause
Data science can be considered a field of science, a concept, and for some a synonym, but in a broad sense requires using methods from multiple disciplines to make sense of and decisions based on data.
Like any multistakeholder effort, which requires a broad range of specialized talents working towards an agreed objective, this is no easy or quick task. And yet, it is also a reality that many industries operate in these days, most notably that of the cybersecurity industry, which draws from a huge pool of expertise including computer science, network engineering, business, policy, security and law enforcement.
Jeffrey is like many other data scientists in that he has experience in the theory and practice of statistical and data analysis. He is an advocate of working with industry-based experts as they understand the “nuances and idiosyncrasies of the data.”
“It is important for either the data scientist to gain experience in the domain they are seeking to work in, and/or work with interdisciplinary teams that include domain experts to guide their analysis and provide context,” says Jeffrey.
The need for context to be blended with theory in both data science and cybersecurity is something that is echoed by others, particularly when it comes to employing automation techniques.
An industry perceived weakness of data science’s multidisciplinary basis is the lack of rigorous scientific methodology. This seems to be a symptom of blending methods and techniques from various scientific and mathematical fields as well as the objectives of industry partners who are concerned about the results over the research itself.
Writing in TechCrunch, data scientist Michael Li notes how data scientists compromising on the scientific process can lead projects astray.
“The most important question in data science is not which machine learning algorithm to choose or even how to clean your data. It is the question you need to ask before even one line of code is written: What data do you choose and what questions do you choose to ask of that data?
“Formulating nontechnical requirements into technical questions that can be answered with data is among the most challenging data science tasks — and probably the hardest to do well.”
Data, data everywhere?
The second biggest challenge for any data scientist revolves around data. To be more specific the availability of data, its quality and how it is handled.
Although the recent decade has given rise to big data in multiple industries, this has not necessarily been the case in cybersecurity. As many cybersecurity professionals will attest to, collecting and sharing of data is not a great strength of the industry — security breaches are still something that many organizations, financial institutions especially, are hesitant to share as reputation is an important asset.
Read: Industry based CERT provides members confidence to share
While your company may have terabytes or petabytes of data to work with — which Jeffrey says can be overwhelming when it comes to knowing what’s relevant or not, remembering that studying network data for malicious activity is still a relatively new concept with few best current practices to reference and still requires a great deal of time and expense to clean and analyse — not being able to compare this to what other networks are seeing, or not seeing, reduces the skill of any automation system.
“As data science generally takes a statistical approach to learning from data, if there are insufficient amounts of data, the conclusions that can be drawn may be biased from the limited data samples,” explains Jeffrey.
Bias, in its many forms, is a major challenge for machine learning projects with a recent survey on the state of data science finding that only 15% of respondents had implemented a bias mitigation solution, and 39% of enterprises surveyed saying they had no plans to address bias in data science and machine learning.
“Quality of the data also matters,” adds Jeffrey. “If the data is noisy and/or has many missing values, then that affects the confidence we have about the analysis and what we can predict.”
Michael Li also touches on this quality issue: “Real-world data is notoriously dirty and many assumptions have to be made to bridge the gap between the data we have and the business or policy questions we are seeking to address. These assumptions are also highly dependent on real-world knowledge and business context.”
Automation and data science are a long-term investment
This is by no means an exhaustive review of the situation and challenges associated with data science in cybersecurity but hopefully builds on the lessons shared in a post we featured earlier this week that automation, and with it data science, cannot promise a quick fix to the cybersecurity problem. Nevertheless, they have the potential to enable security operation centres to react and/or mitigate faster than they do today, if given the time and resources to be properly trained and integrated into an organization’s security framework and policies.
If you or a colleague is a data scientist studying and/or working in the cybersecurity industry we’d love to hear from you in the comments below and welcome any follow up story ideas and contributions.
The views expressed by the authors of this blog are their own and do not necessarily reflect the views of APNIC. Please note a Code of Conduct applies to this blog.