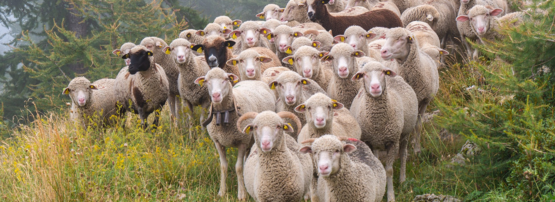
Correctly mapping Autonomous Systems (ASes) to their owner organizations (also called AS2Org mapping) is crucial for Internet researchers. It connects AS-level research and real-world Internet events. For instance, in an Internet censorship event, accurately identifying ASes of government-controlled organizations is the key to discovering which networks conducted censorship (including blocking social media, shutting down mobile services, and so on) and further, estimating those affected.
In this context, organizations can be classified as either single-AS or multi-AS based on the number of ASes they operate. In particular, the inference of sibling ASes (ASes operated by the same organization) is the prime result of AS2Org mappings of multi-AS organizations. Sibling ASes are important as their organization is more likely to play a critical role on the Internet. However, two commonly used data sources, whois databases and the CAIDA AS2Org dataset (CA2O) contain many inaccurate mappings that lead to inaccuracies in sibling relationships.
Our team, the Internet Intelligence Research Lab at Georgia Tech, is working on solving this problem. We have made progress in understanding two systematic root causes of the inaccuracies (please refer to our paper Improving the Inference of Sibling Autonomous Systems), and we have published an improved dataset with more accurate inferences of sibling ASes.
To what extent does whois data impact CA2O inferences?
CA2O is a structured dataset specialized for AS2Org mappings, including inferences based on whois data. Because whois data is the sole source of information, CA2O makes highly consistent inferences compared to whois mappings. Out of 99,271 ASes, only 829 (0.8%) fall into the differences between the two data sources. Other than some manual updates made by CAIDA, most of the differences come from CA2O grouping some ASes into families based on the commonalities of whois fields (for example, phone).
Such a high degree of similarity leads to two conclusions. First, CA2O considers orgID as the only identifier of organization objects. An orgID is an identifier of organization objects linking ASes to organizations, stored in different fields in different whois databases: aut.org / org.organisation fields in APNIC, RIPE, and AFRINIC whois; org.Handle in ARIN whois; aut.ownerid field in LACNIC whois. Second, inaccuracies in whois databases have cascading effects on CA2O inferences. Thus, we are focusing on revealing the root causes of whois inaccuracies.
Two types of inaccuracies on sibling relations and their root causes in whois data
Our research has revealed two types of inaccuracies with sibling relations in whois data: incorrect sibling relations (root cause: APNIC LIR Issue) and missing sibling relations (root cause: Multi-orgID Issue).
Incorrect sibling relations and the APNIC LIR issue
In this type of inaccuracy, ASes operated by different organizations are incorrectly inferred to be sibling ASes due to the same orgID. For instance, for three ASes (AS9426, AS23895, AS45228) owned by Westpac Bank, CA2O incorrectly maps them to Singtel Optus. Consequently, they are inferred to be siblings together with other 60 irrelevant ASes.
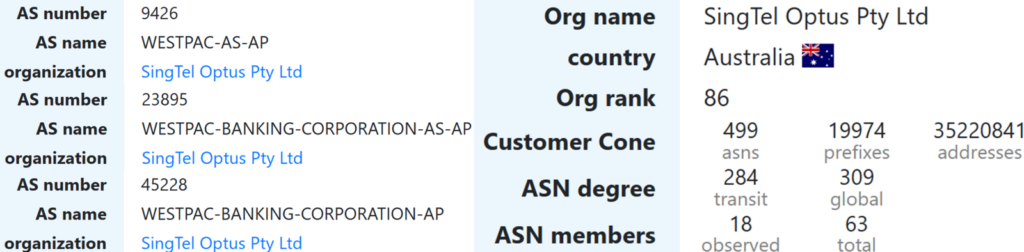
The root cause behind this is a special policy of Internet resource allocation in the APNIC region. Different from other regions, Local Internet Registries (LIRs) in APNIC are responsible for applying AS numbers on behalf of their customers (non-APNIC account holders). As APNIC considers LIRs as resource holders of these ASes, LIRs maintain whois data and include their orgIDs in the aut.org field, while putting the actual resource holder’s name in the description field. Because CA2O regards orgID as the only identifier of organization objects, customer ASes of APNIC LIRs (operated by different end users) are incorrectly inferred as sibling ASes by CA2O.
Note: APNIC is currently addressing this issue via the ‘ASN Delegation Identity’ project, which is scheduled for release by the end of March. See the APNIC Product Roadmap for more details.
Missing sibling relations and the Multi-orgID Issue
In this type of inaccuracy, CA2O is not able to discover all sibling ASes owned by one organization but infers them to different organization objects. For instance, there are three organization objects in CA2O with the exact same name Amazon.com, Inc., while each of them only contains a part of seven sibling ASes of Amazon.
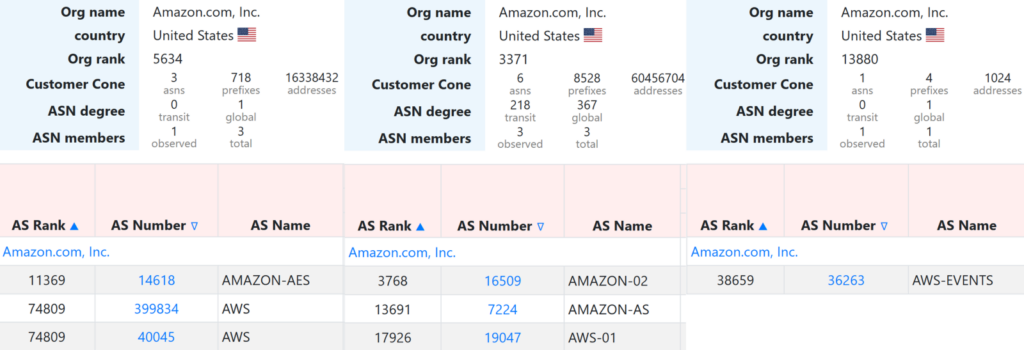
The root cause, Multi-orgID Issue, refers to organizations using more than one orgID for their ASes, which is a universal problem among all five RIRs. There are various reasons behind this, such as the use of multiple orgIDs for the subsidiaries and working groups, the need of registering ASes under different RIRs, and acquisitions and mergers.
Towards an improved AS2Org dataset on sibling ASes
We have generated an improved dataset on sibling relations. It includes corrected inferences related to 1,028 CA2O organizations and 3,772 ASNs, which is equal to 12.5% of organizations with sibling ASes in CA2O. Among these ASes, 15% are affected by incorrect sibling relations due to the APNIC LIR Issue, and the rest are affected by missing sibling relations due to the Multi-orgID Issue.
We have designed and implemented an automatic approach to update the improved dataset. We are planning to update the dataset after every new release of CA2O to benefit the research community.
For further information on the research team’s work, read their paper, “Improving the Inference of Sibling Autonomous Systems” as accepted at the Passive and Active Measurement Conference 2023.
Zhiyi Chen is a PhD student in Computer Science at the Georgia Institute of Technology, specializing in machine learning and Internet measurement.
The views expressed by the authors of this blog are their own and do not necessarily reflect the views of APNIC. Please note a Code of Conduct applies to this blog.