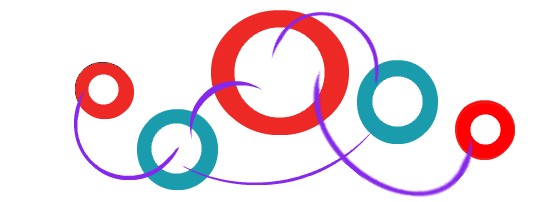
Regional connectivity is not an easy metric to measure, as to do it properly you require measurements generated by multiple vantage points, located in as many places as possible (both geographically and logically). Besides, connectivity is not something strictly defined, as it has no standard definition (as opposed to many metrics we use in today’s Internet).
RIPE Atlas is a great option where it has a considerable amount of probes. But what to do when RIPE Atlas is not enough?
For measuring connectivity in the Latin America and the Caribbean (LAC) region, we first had to define what connectivity really means to us and then find a way to perform distributed large-scale measurements.To accomplish this, we used a worldwide platform, composed of thousands of virtual probes (which are easily deployable), called Speedchecker. Based on the measurements collected, we analyzed a network graph and grouped the data into country clusters.
I presented on the progress of this work at a Lightning talk at APNIC 40 in Jakarta. This post describes the updated results and the method we followed to get our results and provides a perspective of what connectivity is for us and in which state it is in the LAC region.
The study
The data
By performing around 200 daily ICMP pings through 12 months, we built a latency dataset that covered 21 LAC region countries. Out of this dataset, we built a network graph which represented the latency relations between the countries. The geolocation information regarding each measurement is taken from Mozilla Geolocation API and Maxmind Lite database.
Each measurement has its own minimum, maximum, average, and median RTT values. Measurements are built by about 10 samples each, distanced sequentially about one second apart between them (in a traditional ping fashion).
Building the network graph
By grouping all the results by country, we built a graph having countries as nodes and measurements as edges.
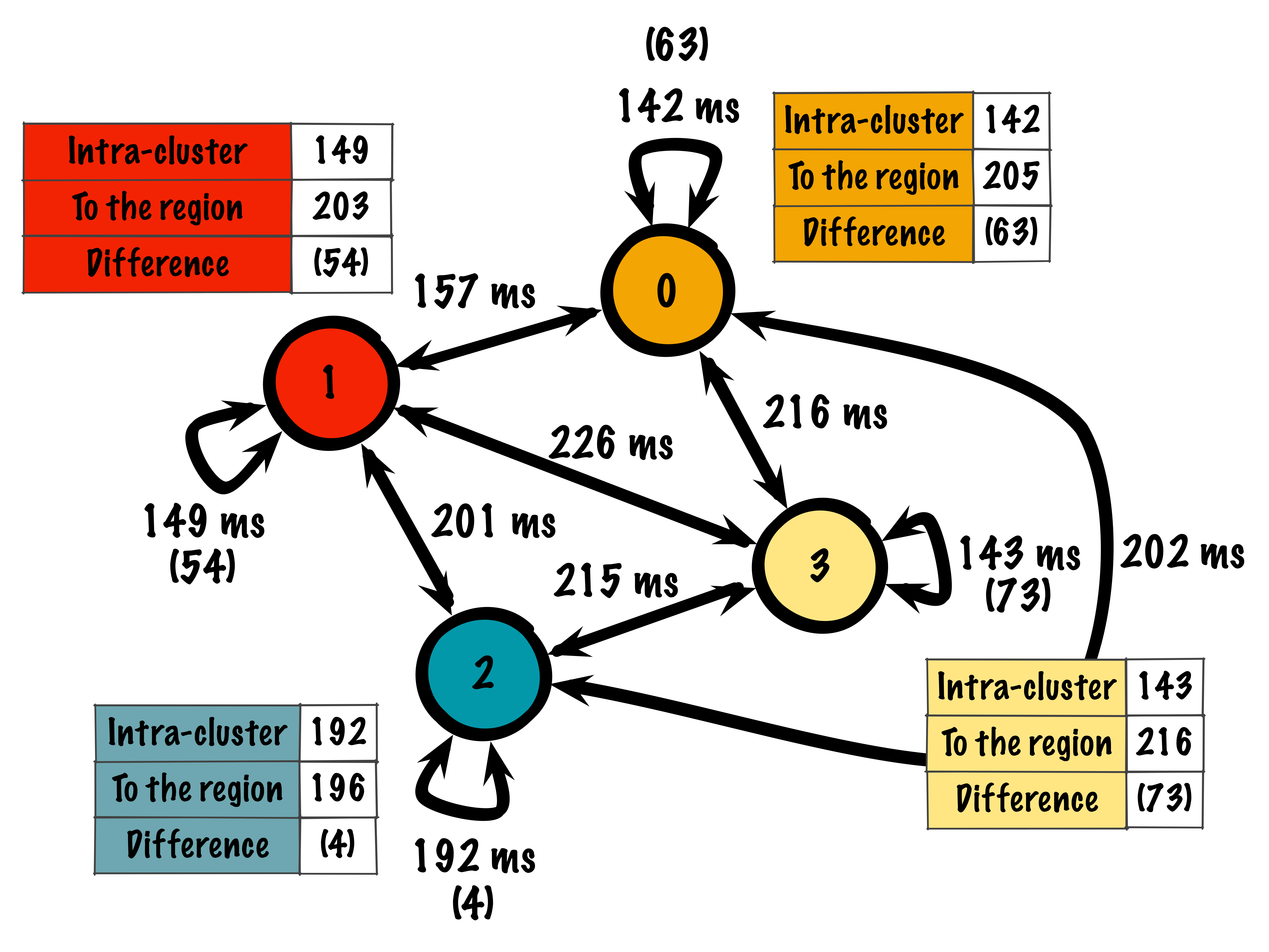
Network graph representing the latency relations between 21 LAC countries.
Cluster 1 (Mexico, El Salvador, Guatemala, Panama, Venezuela)
Cluster 2 (Bolivia, Chile, Peru)
Cluster 3 (Argentina, Brazil, Paraguay, Uruguay)
Cluster 0 (Belize, Colombia, Costa Rica, Cuba, Ecuador, Honduras, República Dominicana, Suriname, Trinidad and Tobago)
The edge connecting nodes A and B is the average minimum measurement taken for that subset of measurements. That means that from the k samples taken between node A and node B, we filter the minimum value for each sample and we take the grand average over that population. As a result, the graph is based on the best latency measurements that could be achieved during the time the experiment was conducted.
Conclusions
Connectivity
The first new insight about connectivity was getting a new definition or at least a more formal approach to the term. Based on our cluster analysis, we could define connectivity based on three metrics: the amount of clusters found by the communities clustering algorithm, the intra-cluster latency, and the inter-cluster latency. We could start building metrics based on these numbers, but having those three metrics already gives a clear view of how close countries and clusters are between them. In the case of the LAC region, four clusters were found. Building new metrics is definitely useful in the cases of having a greater amount of clusters (and therefore an even greater amount of edges).
In the case of the LAC region, we can see that:
- Cluster #2 (Chile, Peru, and Bolivia) has weak intra-cluster connectivity, almost the same as its average inter-cluster connectivity. That means that latency between these countries is not clearly better than to other countries; in this case operators from those countries should start thinking about getting better intra-cluster connectivity, or choose to join another cluster. At a glance, we can say that region two has the weakest connectivity of all.
- Additionally, by looking at cluster #3, we can see that it has good intra-cluster latency values, but high inter-cluster ones. This means that the countries belonging to this cluster (Argentina, Brazil, Paraguay, and Uruguay) have good connectivity between them, but still need to get the cluster closer to the region (cluster #3 is at ~215 ms from the rest of the clusters).
- Finally, clusters #0 and #1 don’t have a clear boundary between them, as their inter-cluster and the edge 0 <–> 1 have very similar values. Looking at the map we see that geographically they are very close and with the geographic boundaries not well defined, as the rest of the cases. That means clusters in Central America and the Caribbean have good ICMP connectivity!
By looking at the graph’s edge values you could draw similar conclusions.
Dis-connectivity
We thought about a way to get a clear view of how bad connectivity links could be detected, as our connectivity graph G only clustered based on good connectivity relationships. Based on the fact that G has its edges (relations) with RTT values, we transformed it in a new graph H, having its edges with 1/RTT values. In this way, by feeding the same clustering algorithm with graph H we could find relationships between countries which are poorly connected between them. Some interesting cases were found, including a couple of neighbouring countries that show poor connectivity between them:
- Argentina and Chile
- Colombia and Venezuela
- Brazil and Peru
Still, despite being neighbours, the pairs of countries mentioned above don’t share the coast between them. The connectivity between these pairs of countries is most likely following well-known “coast” cables, which connect countries belonging to the same coast. That means that part of its traffic is being routed somewhere far and that direct connections are not present or are not being used.
Some final questions
This study leaves us with some open questions regarding connectivity.
- Is there one index that could summarize country-level connectivity health, based on our measurements?
- Do you see any physical connections that are not being fully used?
- Are there any links that are escaping our measurements platform?
- Are software probes worth using (I think they are, especially in regions that are under-represented in mainstream platforms such as Atlas or M Lab)?
Please comment!
Original post appeared on LACNIC Labs
Agustín Formoso works as a software engineer at LACNIC, the Regional Internet Registry for Latin America and the Caribbean.
The views expressed by the authors of this blog are their own and do not necessarily reflect the views of APNIC. Please note a Code of Conduct applies to this blog.